Due to recent technological developments, it is now possible to track our movements at a high level of detail and with relatively low effort and cost, e.g., by using built-in GPS-receivers of our smartphones. This novel data source provides exciting new possibilities for increasing the sustainability of our mobility behaviour through monitoring as well as real-time regulation and management of our transport systems. For instance, there are now innovative systems which aim to monitor and directly influence our mobility decisions by providing eco-feedback (e.g., GoEco!). Such systems rely on identifying when and how their users change their behaviour, and e.g., form more sustainable travel habits. This time-consuming and cost-intensive task is currently mostly done manually. In general, however, methods to automatically detect and evaluate behaviour change are needed for understanding how people will react to new mobility options such as electric vehicles, the shared economy or mobility as a service (MAAS).
In this study, we propose a concept for a fully automated system which continuously monitors movement behaviour based on a stream of movement data, and uses data mining techniques to detect behavioural anomalies.
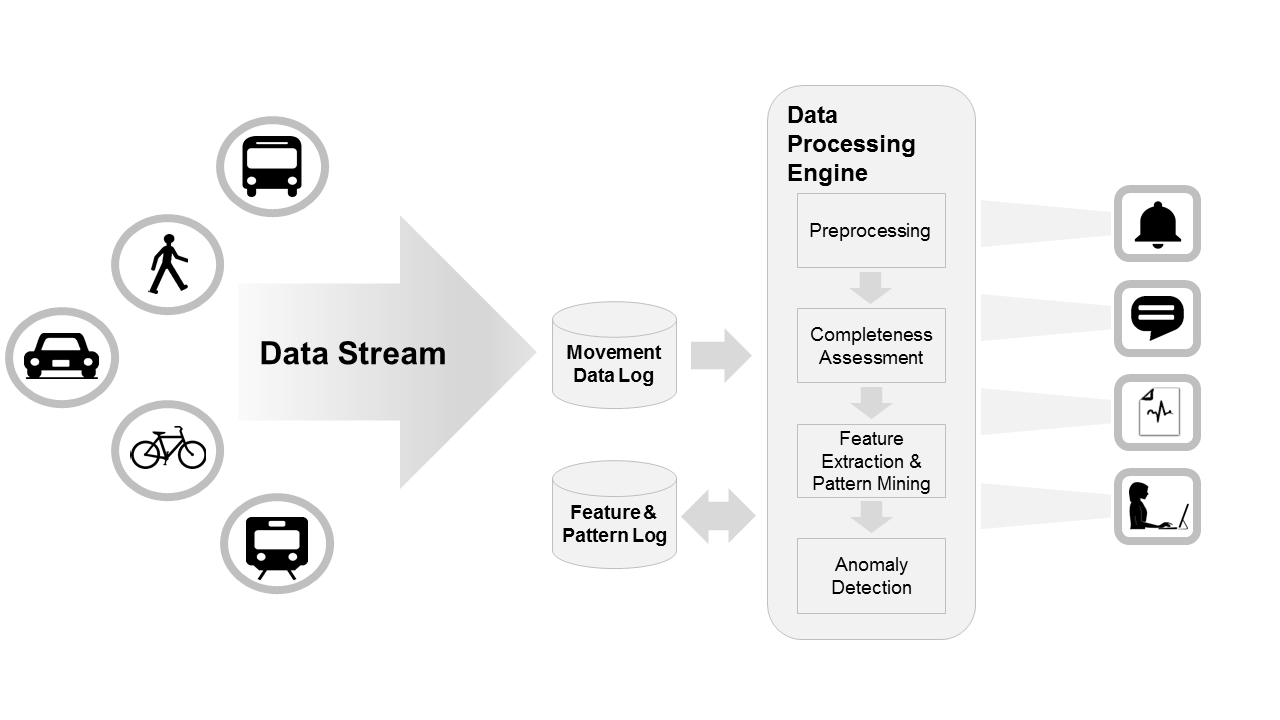
A particular problem here is data incompleteness caused by people not using the tracking application continuously. As these may trigger false alarms, we have to identify and filter these incomplete records in a separate step (Completeness Assessment). Another problem are behavioural changes which are not caused by changing travel habits, but a different contextual situation (e.g., a holiday trip). By additionally comparing the places which are visited by a person, our system is able to filter those temporary anomalies.
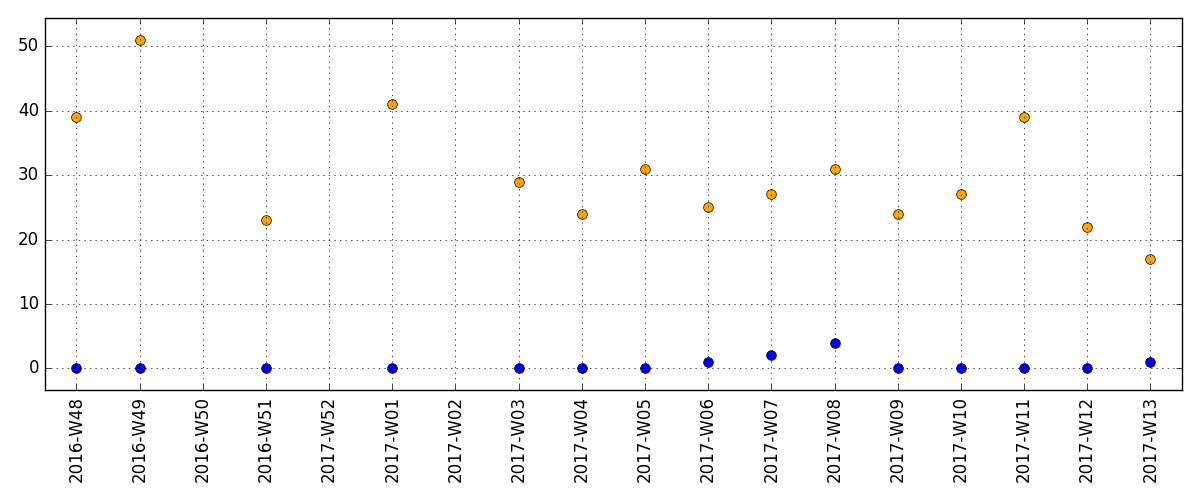
The figure shows the mobility behaviour anomalies (blue) and the place-related (yellow) anomalies for a user of our test sample. Behavioural anomalies are detected from calender week 2017-06 onwards. Since the visited places remain the same, we can conclude that this user indeed changed her mobility behaviour habits. In the future, a fully automated system could interpret and automatically react to this behaviour change, e.g., by sending out notifications to the users or analysts, triggering a response (e.g. encouraging or discouraging the observed behaviour change), logging the occurrence of the anomaly in a database, or providing information to an expert for decision support.
For more information, see
Jonietz, D., Bucher, D. (accepted): Continuous Trajectory Pattern Mining for Mobility Behaviour Change Detection. Accepted at: LBS 2018, Zurich, Switzerland.