On March 30th the kick-off event for the project “An Open Digital Twin Platform for Research on the Swiss Mobility System” (ODTPR-SMS) took place at the LEE building together with our research partners at the Institute for Transport Planning and Systems, the Swiss Data Science Center and the Center for Sustainable Future Mobility. In the latter, the Geoinformation-Engineering group is a key stakeholder. The project is part of the Swiss National Strategy and Action Plan for Open Research Data and funded by swissuniversities through a Swiss Open Research Data Grant and has been acquired by our lab member Jascha Grübel. ODTPR-SMS has been funded with 1.5 million CHF (matched funding) to develop an Open Digital Twin Platform to underpin development on a mobility-specific Digital Twin “CH on the move”. In the kick-off meeting, all involved parties agreed on the timeline, distributed tasks, discussed the licensing of the software and got to know each other. Everybody was thrilled to contribute to strategically important tool chain that will be developed in the coming 24 months. As part of the project, 2 postdocs will join us at the Center for Sustainable Future Mobility to develop key features of the system.
The Open Digital Twin Platform (ODTP) is bringing together cloud computing, data semantics, licensing, access control and visualisation to enable a holistic processing of data covering data acquisition, data representation, data processing, data analysis and data visualisation. Known tools for mobility research can be automated within ODTP to provide (micro-)services and apply to new scenarios with less overhead than ever before. ODTP takes care of the provisioning and deployment of software allowing researchers to focus on their scientific questions rather than how to get the technology working. To quickly assemble a new Digital Twin, ODTP makes use of a “Digital Twin Zoo” that hosts key software as containers readied to be micro-services. Throughout our project ODTPR-SMS, we will develop a first set of mobility related micro-services that include well-known tools such as MATSim, eqasim and our own trackintel. We are also making available as many data sources on Swiss mobility as possible starting with the National Data Infrastructure for Mobility (NaDIM).
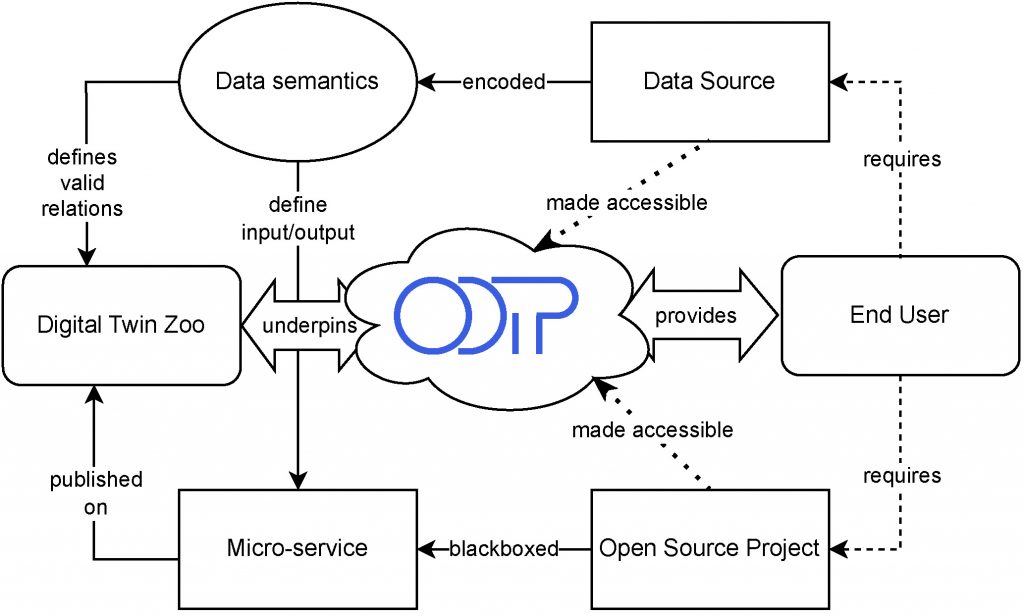
Figure: Overview of ODTP for end users. ODTP facilitates access to open-source software and data through a container-based backend and hosting infrastructure.
ODTP will be accompanied by an open standard for digital twinning. We are looking for experts to evaluate our platform and if you are interested in mobility, digital twins or a combination thereof, please contact Jascha Grübel (jgruebel@ethz.ch) for details on how to join our evaluation board which will be published at the Center of Sustainable Future Mobility’s website. We are also looking forward to add more mobility related software tools to ODTP and we are interested to discuss potential new services, again please contact Jascha Grübel for more details (jgruebel@ethz.ch).
Reference: Grübel, J., C. Vivar Rios, M. Balać, Y. Xin, R. M. Franken, S. Ossey, M. Raubal, K. W. Axhausen and O. Riba-Grofnuz (2023a) “CH on the move”: Introducing the Prototype Digital Twin of The Swiss Mobility System, paper presented at the Swiss Transport Research Conference 2023.