Our paper “GeOT: A spatially explicit framework for evaluating spatio-temporal predictions”, is now published in the International Journal of Geographical Information Science (IJGIS)!
Motivation: While the GIS mantra “spatial is special” is widely acknowledged, it has not made its way into how we evaluate spatio-temporal predictions. Standard error metrics like MSE or MAE are typically averaged over locations, ignore the spatial structure of the data.
Contribution: To close this gap, we propose to leverage Optimal Transport (OT), a theoretical framework to compare two distributions. With OT, we can measure the difference between the true and predicted spatial distribution by computing the minimal transportation costs to align them.
Results: We show that our GeOT framework 1) effectively measures the spatial quality of predictions, 2) relates to spatial autocorrelation, and 3) provides interpretable results. On top of that, this approach is not just a new spatial evaluation metric – it can also be integrated as a loss function in machine learning tasks.
Check out our paper and code base with tutorials.
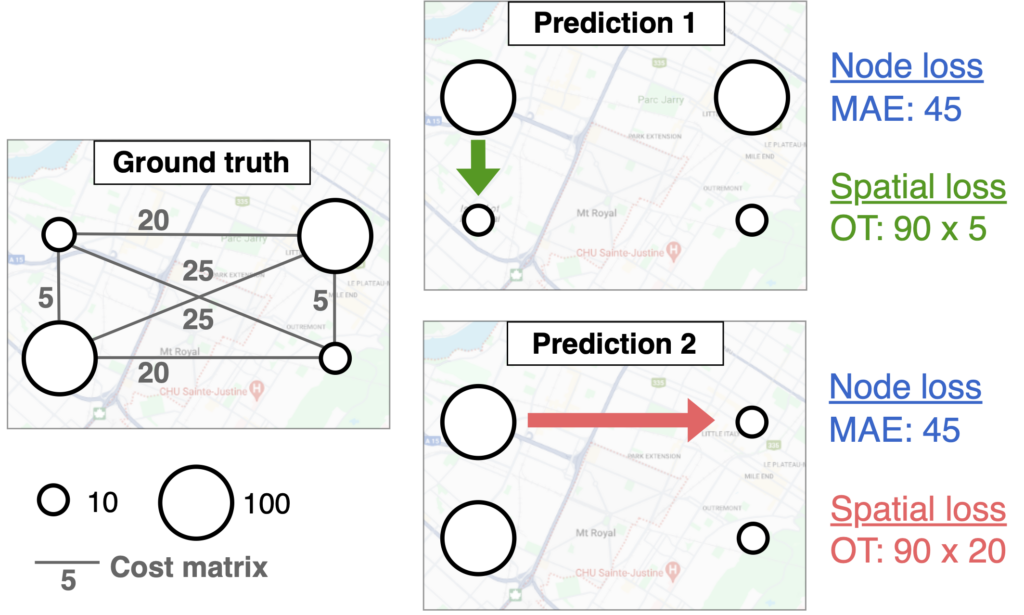