Our new paper, “Rethinking Bikeability Indexes: Fusing Knowledge Graph and MCDA Technique for Multi-criteria Bike Network Evaluations,” was presented by Ayda Grisiute at the 28th AGILE Conference on Geographic Information Science, 10–13 June 2025. It introduces a novel method for evaluating bike infrastructure by integrating a curated knowledge graph of bikeability metrics with the Analytic Network Process (ANP), a decision modelling technique.
The study addresses limitations in current bikeability indexes, which often oversimplify how different metrics interact. By combining over 270 metrics and 41 qualitative criteria from global studies with ANP’s ability to model interdependencies, our approach offers a more rigorous and transparent way to evaluate cycling networks. Applied to Zurich’s road network, the method produces a segment-level bikeability index and includes sensitivity analysis to assess the robustness of results under structural changes.
Check out our paper and the corresponding code on Github!
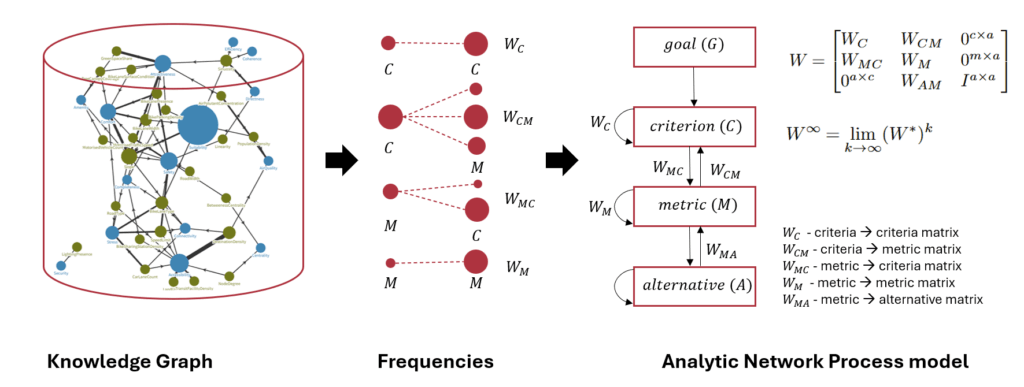