In our recently published 2021 GIScience paper “Estimation of Moran’s I in the Context of Uncertain Mobile Sensor Measurements“, we study the problem of how to quantify the spatial autocorrelation of samples that were recorded at different points in space and time. Such data is commonly produced by mobile sensors, e.g., the temperature sensors of cars, but also by social phenomena such as location checkins of people. As not only the sensors move, but also the phenomena (potentially) change over time, an autocorrelation value such as Moran’s I can either be inaccurate or impossible to compute due to a lack of data. Instead, we propose to weigh the contribution of different samples based on an empirically projected uncertainty. This weakening of the impact of uncertain samples leads to more stable estimations of spatial autocorrelation quantifications such as Moran’s I.
Continuous Trajectory Pattern Mining for Mobility Behaviour Change Detection
Due to recent technological developments, it is now possible to track our movements at a high level of detail and with relatively low effort and cost, e.g., by using built-in GPS-receivers of our smartphones. This novel data source provides exciting new possibilities for increasing the sustainability of our mobility behaviour through monitoring as well as real-time regulation and management of our transport systems. For instance, there are now innovative systems which aim to monitor and directly influence our mobility decisions by providing eco-feedback (e.g., GoEco!). Such systems rely on identifying when and how their users change their behaviour, and e.g., form more sustainable travel habits. This time-consuming and cost-intensive task is currently mostly done manually. In general, however, methods to automatically detect and evaluate behaviour change are needed for understanding how people will react to new mobility options such as electric vehicles, the shared economy or mobility as a service (MAAS).
In this study, we propose a concept for a fully automated system which continuously monitors movement behaviour based on a stream of movement data, and uses data mining techniques to detect behavioural anomalies.
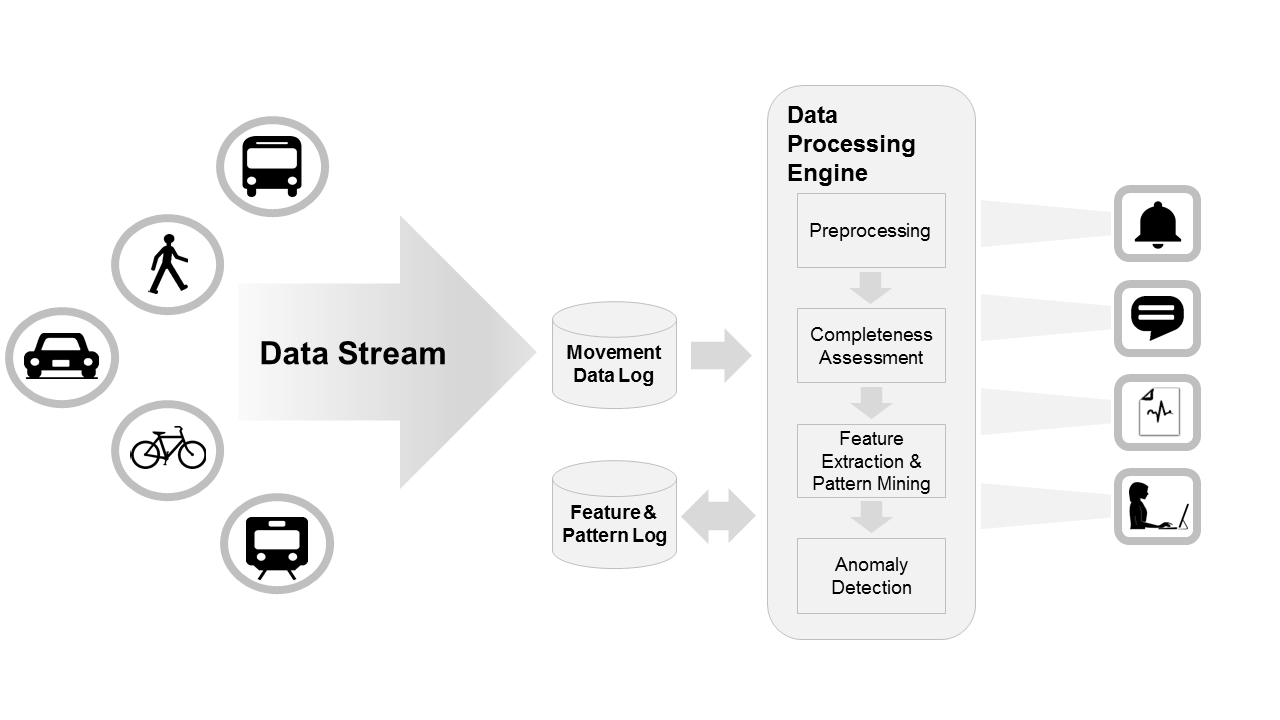
A particular problem here is data incompleteness caused by people not using the tracking application continuously. As these may trigger false alarms, we have to identify and filter these incomplete records in a separate step (Completeness Assessment). Another problem are behavioural changes which are not caused by changing travel habits, but a different contextual situation (e.g., a holiday trip). By additionally comparing the places which are visited by a person, our system is able to filter those temporary anomalies.
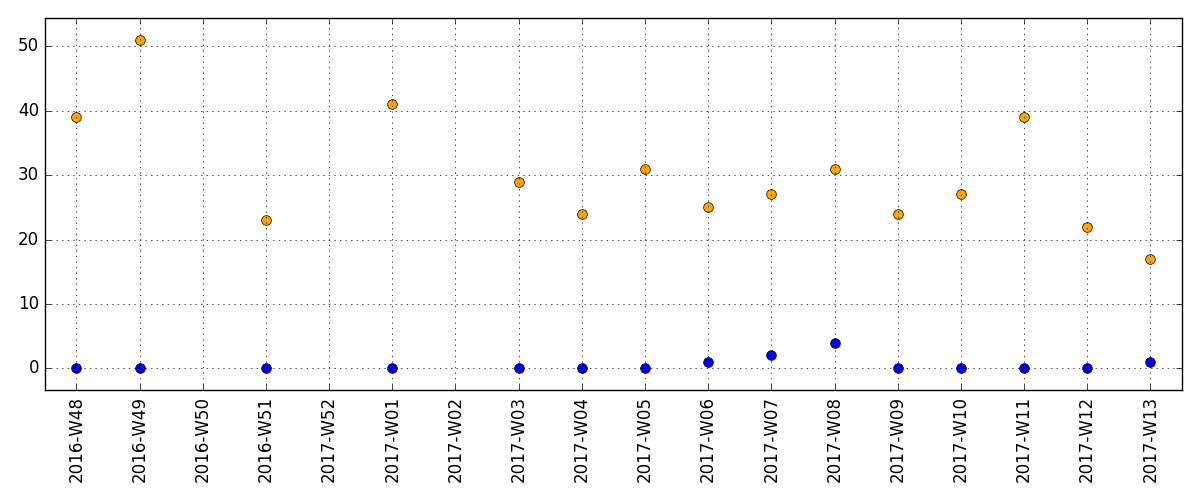
The figure shows the mobility behaviour anomalies (blue) and the place-related (yellow) anomalies for a user of our test sample. Behavioural anomalies are detected from calender week 2017-06 onwards. Since the visited places remain the same, we can conclude that this user indeed changed her mobility behaviour habits. In the future, a fully automated system could interpret and automatically react to this behaviour change, e.g., by sending out notifications to the users or analysts, triggering a response (e.g. encouraging or discouraging the observed behaviour change), logging the occurrence of the anomaly in a database, or providing information to an expert for decision support.
For more information, see
Jonietz, D., Bucher, D. (accepted): Continuous Trajectory Pattern Mining for Mobility Behaviour Change Detection. Accepted at: LBS 2018, Zurich, Switzerland.
News Article in NZZ about SBB Green Class
On the 21st of October, the NZZ reported about the planned one-year extension of the project SBB Green Class, for which we provide the lead scientific support. In the article, Prof. Dr. Martin Raubal reports on selected preliminary findings.
Best Paper Award Nominee at Energieinformatik 2017
Our paper “Using Locally Produced Photovoltaic Energy to Charge Electric Vehicles” shared the second place for best paper with a contribution from the University of Bamberg / LAGRAR at D-A-CH+ Energieinformatik 2017.
You can find more information about the paper in our previous post and on our publications site.
Best Poster Nomination at the 4th SCCER Mobility Conference
The poster “Extracting Eco-Feedback from Movement Trajectories“, which we presented in collaboration with our colleagues from SUPSI, was nominated for the Best Poster Award at the 4th annual SCCER Mobility Conference in Zurich, Switzerland.
Over 120 participants from research and industry attended the conference and discussed relevant research topics and projects from the transport and mobility domain. Andreas Lischke (DLR Berlin) and Michael Frambourg (Volkswagen AG) held keynotes on the future of heavy-duty freight transport as well as future plans towards more sustainable passenger cars.
Both speakers emphasized the importance of an (economic and scientific) investement in alternative fuels next to pushing battery technologies forward. This point was later again highlighted, within the context of the rising shares of CO2 emissions from flight travels. With respect to Switzerland, three key topics were discussed in particular: 1) the logistics challenge, 2) passenger mobility in cities and regions, and 3) energy for transportation.
Interview with Prof. Martin Raubal on the Project SBB Green Class
Prof. Martin Raubal has answered questions regarding our data analyses in the course of the SBB Green Class Project. The full interview (in German) can be found here.
Using Locally Produced Photovoltaic Energy to Charge Electric Vehicles
As more and more countries announce their plans for banning combustion engines (e.g., [1, 2]), it becomes critical to find ecologically sustainable ways to power electric vehicles. While wind and water provide energy throughout the whole day, photovoltaic installations have production peaks around midday which need to captured either by smart appliances (such as washing machines) or by some form of energy storage, such as batteries or water reservoirs. A solution for both problems could be to use the car batteries to cap the solar production peaks – possibly even completely locally, i.e., without requiring transmission lines to transport energy over large distances.
Our paper Using Locally Produced Photovoltaic Energy to Charge Electric Vehicles [3] (to be published in Computer Science – Research and Development and presented at D-A-CH+ Energieinformatik 2017) discusses this potential of using solar energy to power electric cars of commuters in Switzerland. On the one hand, we compute the potentially available solar energy on rooftops in each municipality:
This is put in relation to the energy required by all work commuting travels, both within municipalities, as well as between different ones. The routes traveled are given as follows:
In a first scenario, we assume that people only charge their electric cars during the day at their workplace. We find that without shifting power from one municipality to another it is possible to cover up to 89% of the energy demand of commuter mobility. This also means that in larger cities (which have a high photovoltaic potential due to many rooftops), energy production peaks can be captured by electric cars, without the need to deploy additional batteries or storage capacity. The following figure shows the energy balance in July:
In a second scenario, where people only charge their cars at home (usually during the night), solar energy could cover 99.95% of the commuter energy demand. However, this requires installed storage capacity of around 9.32 GWh to shift the energy from day to night. Currently, 13.5 kWh batteries are targeted at homeowners, which means that around 690’000 households in Switzerland would need to be equipped with such a battery to let people charge their cars at home during the night.
The above presented analyses consider a full coverage of rooftops with solar panels, as well as a complete replacement of combustion engine cars with electric cars. This shift will not happen instantaneously, so the values should be taken solely as indications of potential future energy balances. It also needs to be noted that leisure travel and freight transport make up a substantial amount of mobility, which is not represented in above numbers. For more details, seasonal influence on solar energy production, and scenarios where people charge cars both at home and at work, please refer to the paper.
[1] https://www.theguardian.com/business/2017/jul/06/france-ban-petrol-diesel-cars-2040-emmanuel-macron-volvo
[2] http://www.independent.co.uk/environment/climate-change/norway-to-ban-the-sale-of-all-fossil-fuel-based-cars-by-2025-and-replace-with-electric-vehicles-a7065616.html
[3] https://www.research-collection.ethz.ch/handle/20.500.11850/173513
Energy-based Routing and Cruising Range Estimation for Electric Bicycles
Personal mobility is critical for successful cities, but also a major threatening factor for the livability they provide to a growing urban population. For this reason, it is highly important to switch towards more sustainable travel behavior, and substitute many of the trips previously covered by car with smaller and more energy-efficient modes of transport, such as electric bicycles. With rising sales numbers, e-bike fleets in companies and emerging e-bike sharing systems, there is a growing demand for services which provide e-bike specific routing and range assessment.
In the MIE Lab, we developed a prototypical GIS-based application with the aim of route planning and cruising range estimation for electric bicycles. The model parameters were either measured during field tests with electric bicycles, or taken from literature and the bicycles’ specifications.
For more information see:
Energy-based Routing and Cruising Range Estimation for Electric Bicycles
Simon Haumann, Dominik Bucher, David Jonietz
In Proceedings of the Short Papers, AGILE 2017, Wageningen, The Netherlands. Best Short Paper Award
Or try out the application on ebikes.dominikbucher.com!