Our paper Bike Network Planning in Limited Urban Space was accepted for publication in Transportation Research Part B: Methodological!
This paper was part of the e-bike city project, a multidisciplinary initiative at the department exploring the effects of a radically changed urban road space with priority on cycling. Planning a suitable bike network is a core challenge in this project. In our paper, we introduce a novel optimization method for placing new bike lanes with minimal impact on other travel modes.
Our approach leverages the concept of Pareto-optimality: When car lanes are repurposed as bike lanes, car travel times inevitably increase. The key question is: By how much? Pareto-optimal solutions are the street networks that present the best trade-off between car accessibility and bikeability. To quantify bikeability, we introduce the concept of “perceived bike travel time”, based on research showing that cyclists perceive dedicated bike lanes as faster. Our experiments show that the optimization approach outperforms other methods by proposing networks that have both lower car travel times and lower perceived bike travel times.
Check out our paper or get in touch if you are interested to know more!
Further resources:
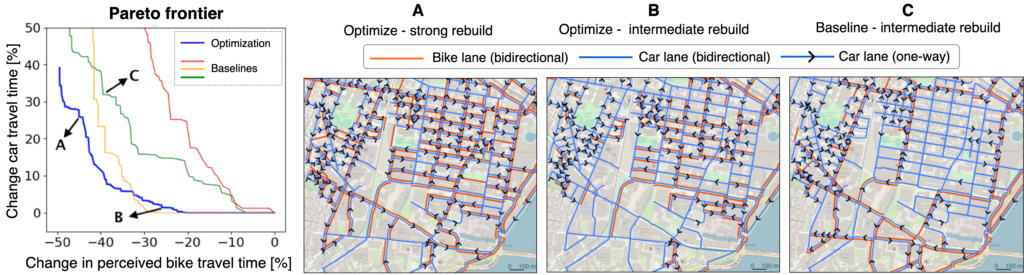