What is the potential for integrating vehicle-to-grid with car sharing in the future? As part of the V2G4Carsharing project, we simulated scenarios for 2030 and quantified the benefits in terms of monetary savings and peak shaving effect. In our case study done in collaboration with the swiss car sharing provider Mobility, we found that Mobility could offer flexibilities between 12 to 50 MW, dependent on the scenario. There is a sweet spot where both car sharing and power grid operators benefit.
Our paper titled Vehicle-to-grid for car sharing – A simulation study for 2030 was now published in Applied Energy! Check out the paper here.
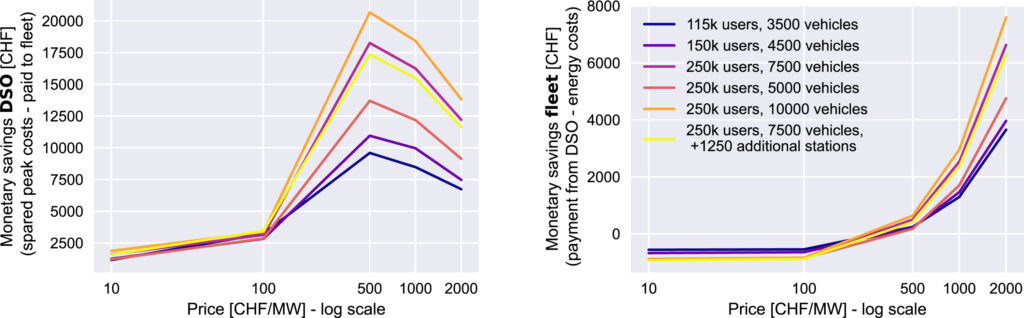
Get in touch if you are interested to learn more, or checkout our project code base and the car sharing simulator.