Our paper on “Quantifying the dynamic predictability of train delay with uncertainty-aware neural networks” has been published in Transportation Research Part C!
In light of the importance of accurate delay prediction for transport services and passengers, many predictive methods have been proposed. However, they hardly account for the involved uncertainty and there is a lack of work analysing the dynamic predictability over time. We fill this gap with an uncertainty-aware neural network and a framework for describing the predictability by the prediction horizon. The results on Swiss train delay data show 1) an exponential decay of the predictability by the horizon, 2) a significant portion of (aleatoric) data uncertainty in contrast to model uncertainty, and clear advantages of the NN compared to MC models.
For more details, check out our paper! This work was done in collaboration with the Institute for Transport Planning and Systems at ETH Zurich.
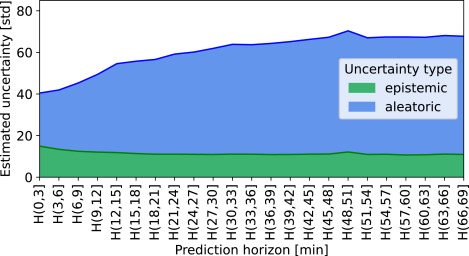