A new paper titled “Enhancing Deep Learning-Based City-Wide Traffic Prediction Pipelines Through Complexity Analysis” was published in the journal Data Science for Transportation.
Our research introduces a novel metric that allows for the pre-modelling complexity evaluation of traffic prediction tasks. This metric, designed for computational efficiency and to be architecture-agnostic, assists in choosing the most suitable model for specific traffic scenarios. It advocates a shift from the traditional “bigger-is-better” approach in deep learning-based traffic prediction to a “just-enough” complexity model, optimizing both performance and resource utilization.
The findings enable a refined approach to modelling traffic prediction tasks, such as deciding between multiple chained predictions and a single-shot prediction. Furthermore, it allows for the segmentation of urban areas into regions of varying complexity, paving the way for deploying ensembles of models that better address diverse urban dynamics.
For more details on this transformative approach and its potential impacts on urban traffic management, check out our paper!
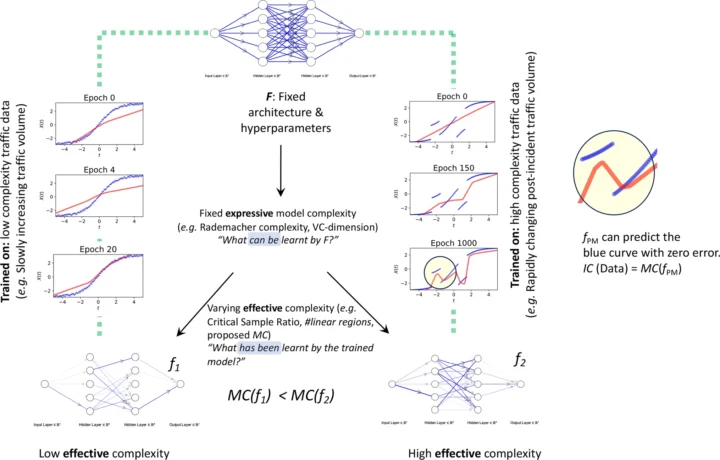